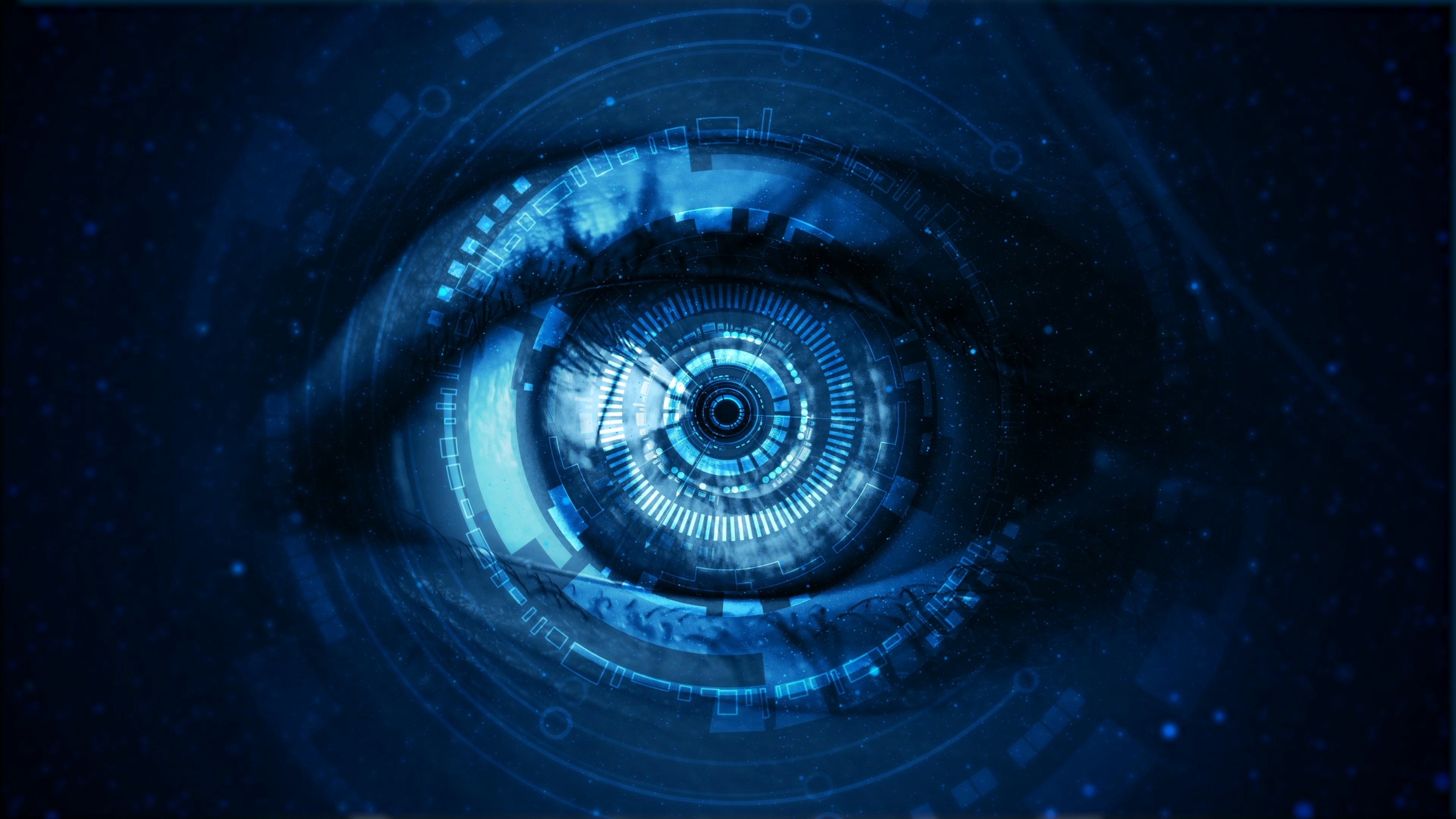
Researchers at UC Santa Barbara have shown that the behavioral markers of covert attention — once thought to be the exclusive domain of primates — may actually be more of an emergent form of intelligence rather than one associated with a particularly evolved brain architecture. Using a type of artificial intelligence model called a feedforward convolutional neural network (CNN), they demonstrated that a relatively simple brain analog without an explicit attention mechanism can demonstrate the main signatures of covert attention in perceptual tasks.
“To some extent, people thought this covert attention business was something of humans, of primates, and that was it,” said Miguel Eckstein, professor of psychological and brain sciences. Some scientists have proposed that attention is related to awareness and consciousness.
“But as years have gone by, the behavioral signatures of covert attention have been shown in animals, such as crows, rodents, archer fish, and even bees. So that motivated us to think that there must be something simpler that gives rise to these effects. And that was the starting point of our paper,” said Eckstein, who with computer scientist William Wang and graduate student researcher and lead author Sudhanshu Srivastava published their work in the journal Current Biology.
Highly efficient information processing
Human visual attention is often conceptualized as a spotlight that scans the visual world or as resources that can be centered on an object or distributed over a spatial region. Covert attention accomplishes all these things, but without moving one’s eyes. It’s an efficient way to quickly gain information from multiple locations simultaneously, as opposed to focusing all attention only on the location you are looking at. Unsurprisingly, response to visual stimuli (performance) is improved where one places their attention than in unattended locations.
“When you’re driving, you’re attending at the visual periphery and you are processing moving cars in the neighboring lane without looking at them,” Eckstein said. “And in some social situations, you might covertly attend to a person without moving your eyes because you don’t want to reveal that you’re actually paying attention to them.” There is also empirical evidence that these covert attention mechanisms are also important before one makes an actual eye movement and looks at a particular object, person or region in the visual world, he added. In laboratory search tasks, investigators often try to have human subjects direct covert attention to a particular location by presenting an arrow or box (cues) that indicates a likely location of the search target.
These covert attention mechanisms, which are typically executed when one is searching for something, were once thought to be exclusive to primates, but have since been shown in other animals, animals that don’t even have the mammalian brain structure (the parietal lobes in the neocortex) that is associated with covert attention. However, because the ways we process information — and in particular how we optimize our attention for accuracy — are difficult to map to neurons, traditional theories of covert attention have existed solely in the realm of verbal hypotheses. More recently, scientists often explicitly incorporate into computational models a covert attention mechanism that changes how the attended visual information is processed.
“But you don’t necessarily have to hypothesize these classic psychological concepts nor build an attention mechanism” Eckstein said. Using a 200,000-neuron (primates have billions) convolutional neural network, the researchers focused on the most commonly used tasks to characterize behavioral signatures of covert attention, including Posner cuing (the ability to shift attention), search set size effects (the effect of distractors on the time needed to locate a target) and contextual cuing (targets in repeated distractor configurations are found more quickly).
“The only thing we do, is we give the images to the network and we train it to try to detect the target as best as it can,” Eckstein explained. “We do not build any explicit attention mechanism in the network, and no concept of limited resources — attention — to pre-determine how information at the cued location is processed. All cues appeared to the neural network at the time the images were presented, with no prior knowledge of cues or contexts.” The CNN was left to “decide” how to prioritize the information given. “And what we found, is that in the process of trying to do the best it can to detect the target, the CNN showed the behavioral signatures typically seen in humans,” Eckstein said. “Targets are more easily detected when appearing at cued locations or locations predicted by repeated distractor configurations." Often, the disparity in performance between attended and unattended locations is interpreted as a reflection of limitations in brain resources across locations or objects, he said, however these results showed that the behavioral signatures of covert attention reflect a consequence of an organism trying to do the best it can to find the target rather than brain resource limitations.
“Of course, neural networks don’t reason about cues and contexts,” Eckstein continued. “This is all an emergent process.” With each iteration, he explained, the CNN automatically adjusts the weight, or importance of the information, based on its rates of error in finding the target, through a process known as backpropagation. Compared to a model called the Bayesian ideal observer (BIO) that has access to all information about cue and context, and thus attains “the highest attainable perceptual accuracy, and has historically served as a mathematically elegant benchmark of human vision,” the neural network’s cuing and context performance, absent all the statistical information the BIO received, was “comparable.”
“The Bayesian ideal observer is a really very beautiful and thorough theory, but it can only be applied to simple tasks that we actually create in the lab for which the statistical properties are known and can be incorporated into the model’s math used to make decisions,” Eckstein said. “It cannot be applied to real-world images.” CNNs can be applied to real-world images and there lies their power. Additionally, he said, neural networks can be better mapped to neuronal populations in brains
The researchers’ proof-of-concept opens up exciting new avenues in the realm of brain sciences, by using theoretical CNN results that hint at potential neuronal properties and circuitry associated with covert attention that have yet to be uncovered, and, importantly, moving from verbal psychological theories and laboratory tasks to models (CNNs) that can better relate to neurophysiological measurements and applied to real-world complex tasks. Eckstein and Wang are deeply interested in the interface of human and machine intelligence, heading a Mind & Machine Intelligence initiative (an initiative made possible by the generous gift of Duncan and Suzanne Mellichamp) to bring together people working at the intersection of AI and the study of the mind.
“Down the road, the idea is that this could provide a new, interesting framework to understand how neuronal properties such as feedback connections or biophysical constraints change the CNN’s performance and training,” Eckstein said.
Sonia Fernandez
Senior Science Writer
(805) 893-4765
sonia.fernandez@ucsb.edu