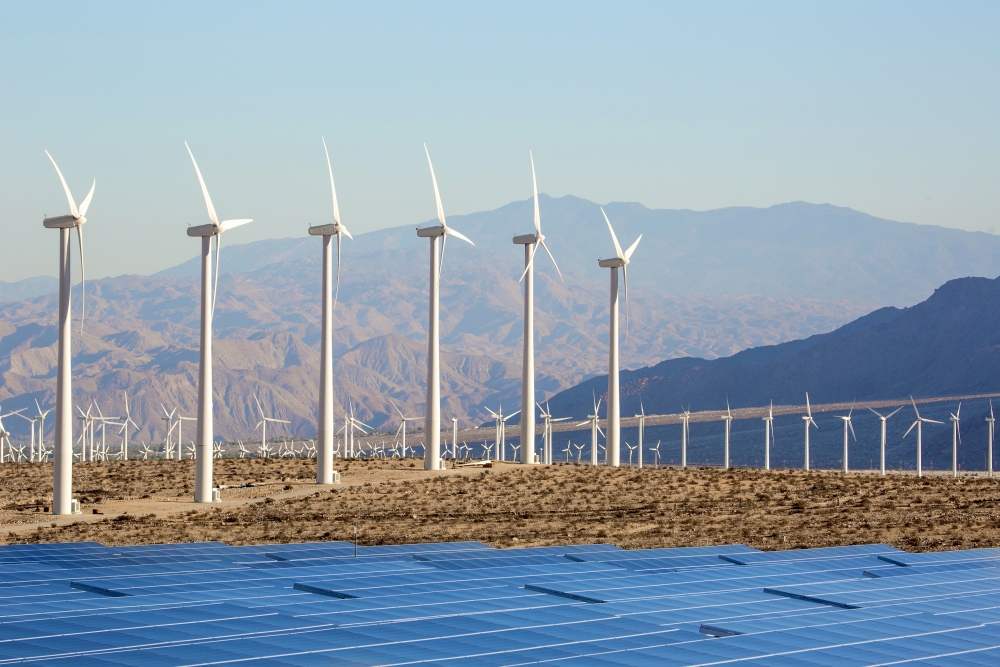
Uncertain Energy
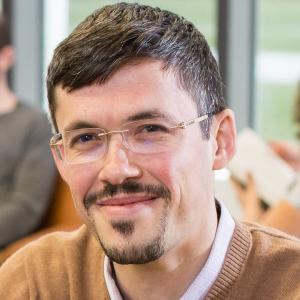
The havoc caused by severe winter storms in Texas last month, and California’s own blackouts during last summer’s heatwaves, vividly illustrate the weaknesses in the United States’ electrical systems. But the country needs to invest in more than just its infrastructure to address the problem, researchers say — the entire electricity market requires a significant update as energy sources shift and the entire sector becomes less predictable.
To tackle this challenge, the Department of Energy has provided $3.5 million through its Advanced Research Projects Agency–Energy (ARPA-E) to study the impact of uncertainty in renewable energy generation on the power grid. Rather than approach the issue from an engineering perspective, the agency has called upon mathematicians, risk managers and statisticians to develop a model that those in the industry can use to better predict the uncertainties involved in renewable energy sources.
Researchers from UC Santa Barbara, Princeton and Scoville Risk Partners (a consulting firm) will team up on Project ORFEUS, short for Operational Risk Financialization of Electricity under Stochasticity. ORFEUS aims to lay the foundations for a more robust, stable and yet nimble energy market in the U.S. This will call for investments in infrastructure, as well as forward-looking legislations and regulations. But planning and oversight also will be necessary, which is where this initiative comes into play.
The project aims to improve how we manage our energy markets so we can account for this new type of energy source: variable renewable energy. It’s one part of the larger effort to create a better electrical system for the expected future, and indeed, the present we already face.
“This is the paradox: Renewable energy is free to generate, and so it sounds amazing. But the fact is there’s always some tradeoff,” said Mike Ludkovski, chair of the Department of Statistics & Applied Probability and UC Santa Barbara’s principal investigator on the initiative.
While renewable energy sources like wind and solar are free to harvest once the infrastructure is there — no coal to mine or gas to extract —the trade-off is a power source that varies from day to day. And unlike, say, a coalfired power plant, a solar farm cannot dispatch more electricity when demand rises.
Solar farms cannot respond to electricity demand like conventional powerplants.
This poses a huge challenge to the ISOs, or independent systems operators, that manage regional grids throughout the country. Since society still hasn’t mastered large-scale energy storage, pretty much all electricity must be generated in real time. ISOs, therefore, have to predict energy demand on an hourly basis every day of the year to ensure the lights stay on.
This was tough but certainly possible when most electricity came from facilities like coal and natural gas plants, which are very reliable and can supply more or less electricity on demand. But our power sources are increasingly shifting to variable renewable energy, introducing new uncertainties into the energy supply.
During midday, the state of California can generate over 50% of its electricity from solar, with plans to expand capacity. “However, going from 50% to the set goal of 100% zero-carbon electricity by 2045 is going to create huge issues in terms of reliability and predictability,” Ludkovski said.
That isn’t to say we shouldn’t do it. The benefits of renewable energy sources have only become clearer in light of the climate crisis bearing down upon the world. It simply means that to avoid a mess, we need to be able to address the challenges of these sweeping changes before we make them.
“Some people come out and say that having 100% renewable energy is just not doable, because it’s going to cost too much and it’s going to create too much instability in the system,” Ludkovski said. “What we’re doing is pushing back on them, saying, ‘you just have to make things more nimble; you have to redesign how we schedule and dispatch electricity generators.’”
Energy demand has also become less predictable, in part due to localized renewable generators like rooftop solar panels. During sunny days, rooftop solar cells provide energy to homes and businesses, reducing the demand for electricity from the grid.
Even with the best weather models, we can’t perfectly forecast how much sun or wind energy we’ll be able to produce on a given day. “The way it works right now is basically if the wind blows, the turbine starts spinning, and the power starts coming out,” Ludkovski said, “and the grid has to react.” Giant batteries might be able to help in the long term, but that likely won’t be the case for at least a couple of decades, he added.
When renewable energy facilities generate more electricity than consumers need, they often have to curtail generation: either shutting off turbines and solar cells or simply running the excess electricity into the ground. Clearly the country’s energy markets could be much more efficient, but we’re still managing them with a 20th century mindset.
Ludkovski, who specializes in risk analysis, and his colleagues aim to create software that can model the inherent uncertainty in renewable energy sources and its effect on the energy market. The package can then be adopted or adapted by ISOs to manage the country’s individual power markets.
The researchers will combine weather forecasts with advanced statistical and probability models to help quantify uncertainty in the energy market. This will yield a statistical prediction engine of how supply and demand might fluctuate within the coming hours, enabling better grid operation and ultimately lower costs to consumers.
Ludkovski said he would love to be able to use the software to provide a prediction like a weather report: “We think there will be 6 GW of wind energy tomorrow at 8 a.m. with an uncertainty of ±100 MW and a 6% chance of an extreme wind event that would send prices soaring.”
Risk analysis and statistics are usually very siloed, but not so at UC Santa Barbara, Ludkovski explained. The university’s Department of Statistics & Applied Probability embraces both fields: “[Many] people in the department are crossing this divide between actuarial science, statistics and quantitative finance,” he said. “So that’s why this proposal was successful, and why I’m really optimistic it’s going to work well.”
ARPA-E’s initial grant will fund 3 years of research and development. Ludkovski plans to use some of the funds allocated to UC Santa Barbara to hire two postdoctoral researchers. If the team is successful, the project will progress to a pilot stage of real-life deployment.