Peering Inside the Black Box
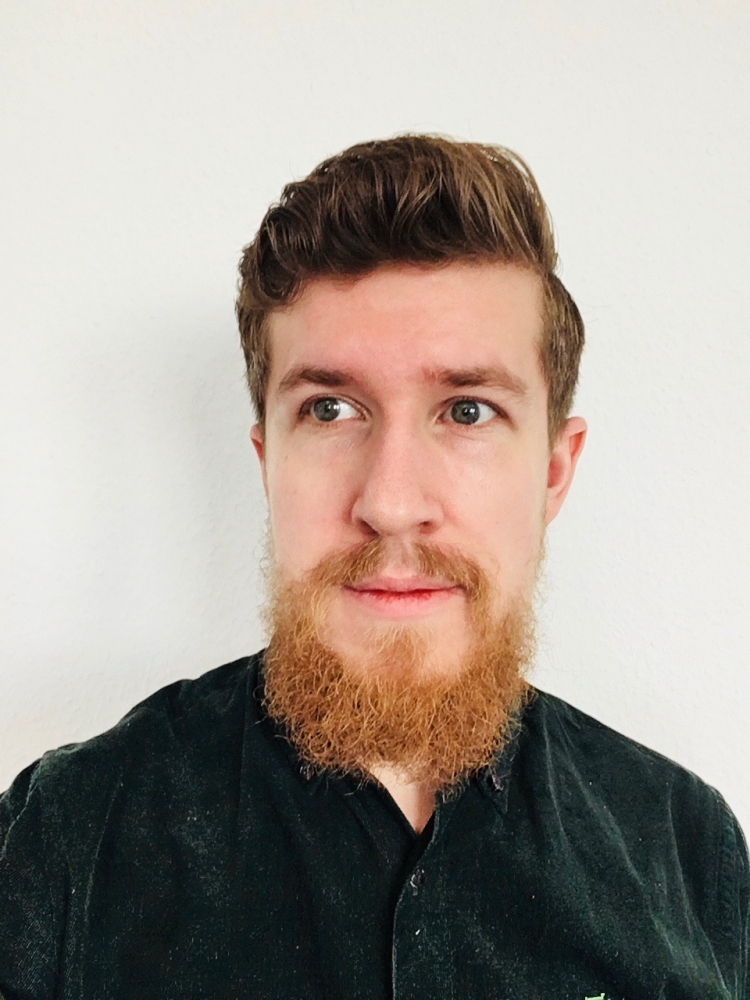
Found in everything from self-driving cars to machine translation, artificial neural networks are currently one of the hottest fields in machine learning.
Now there’s a growing interest in unraveling how these brain-like systems think, and it is providing unexpected insights into our own way of understanding the world. Fabian Offert, a doctoral student in UC Santa Barbara’s Media Arts and Technology graduate program, has brought a scholar’s perspective to this field so often dominated by scientists and engineers.
Before joining UC Santa Barbara, Offert served as a curator at the ZKM | Center for Art and Media in Karlsruhe, Germany. He realized there that this work on neural networks provided a unique opportunity to explore artistic and philosophical concepts. “Their perspectives might be fundamentally irreconcilable with our own,” said Offert, “but I think that’s exactly why this is so interesting, and why people, specifically in the humanities — who deal with interpretation, perception, abstraction and representation — should look at these things.”
Artificial neural networks take inspiration from their biological counterparts. As in a human brain, data streams into the system, where it’s processed by layers of interconnected functions called neurons. Each neuron looks for a particular mixture of features, with those in earlier layers generally picking out lower-level features — like shapes, patterns and colors. Higher layers respond to combinations and relationships between the more basic elements. A long, vertical object between two circles might trigger a set of neurons that recognize faces, for instance. The last layers provide high-level classifications, whereupon the program spits out a result.
Scientists and engineers are keen to understand the processes at work in these neural nets because they often perceive the world in different ways than we do. And in some cases it’s important to know exactly why the network came to a certain conclusion. For instance, when predicting recidivism rates or credit approvals.
To peer inside these black boxes, Offert uses an approach called feature visualization. He takes a neural net trained to classify images and feeds it random noise. But instead of running the entire program he stops on a particular neuron or layer of interest and observes how activated it is. This tells him how far off the noise is from an optimal image for this layer. He then back-propagates the result, tweaks the input and repeats the process until the activation levels plateau. The results provide a surreal approximation of what that particular neuron or layer is really looking for.
The characteristics of an umbrella, according to a neural network.
Photo Credit: FABIAN OFFERT
Sometimes the features correspond with our own experiences. For instance, a neuron activated by images of bees appears to zero-in on alternating yellow and dark stripes. However, sorting out pictures with umbrellas proves more nuanced. This neuron seems to focus on drooping shapes, but is also activated by figures and cool colors.
Some image classes are more difficult to classify, so the ambiguity persists even in the higher levels. “So higher levels won’t just represent one thing, but often a mixture of different things,” Offert said. “And they will serve a mixture of different functions.” By embracing these ambiguous images, Offert argues that scholars can use them to discover properties of images that may not be as intuitive.
In fact, “a single neuron is maybe not the right metric for human interpretability,” Offert suggested, “which is interesting, because it means that, as an artificial perception, the neural network has a very different perspective on the world than we have.”
For example, Offert has looked at the work done by UC Davis computer scientist Gabriel Goh. In 2016, Goh decided to use feature visualization on a neural network that classifies images based on whether or not they’re explicit. As Offert notes, the resulting images are definitely not safe for work, but it’s hard to determine exactly why.
However, Offert was far more interested in the minimally activating images — the most safe-for-work images the system could generate. Since explicit imagery doesn’t have a perfect counterpart, he expected to see just noise. However, a clear trend emerged among these SFW images: Most of them look like cliffs or dams.
Perplexing though this may be, Goh has developed a hypothesis that Offert believes is likely true. Goh thinks that the programmers who trained the network probably used images of cliffs as negative examples to help the system work better.
Cases like this illustrate how fruitful the topic of neural network interpretability can be for scholars in the humanities. “This technique tells you the weird and strange perspective the machine has on the world, but it also tells you the perspective of the people who built the machine, and what they wanted to do with it,” Offert said.
It also forces us to re-examine concepts like representation, abstraction and even the notion of an image itself. But unlike in more traditional musings, in this case these concepts have concrete, technical applications. The bizarre categories that neural networks create actually function and produce a coherent output.
“People in the humanities should be interested in the strange notions of representation that you can extract from these techniques,” Offert said.