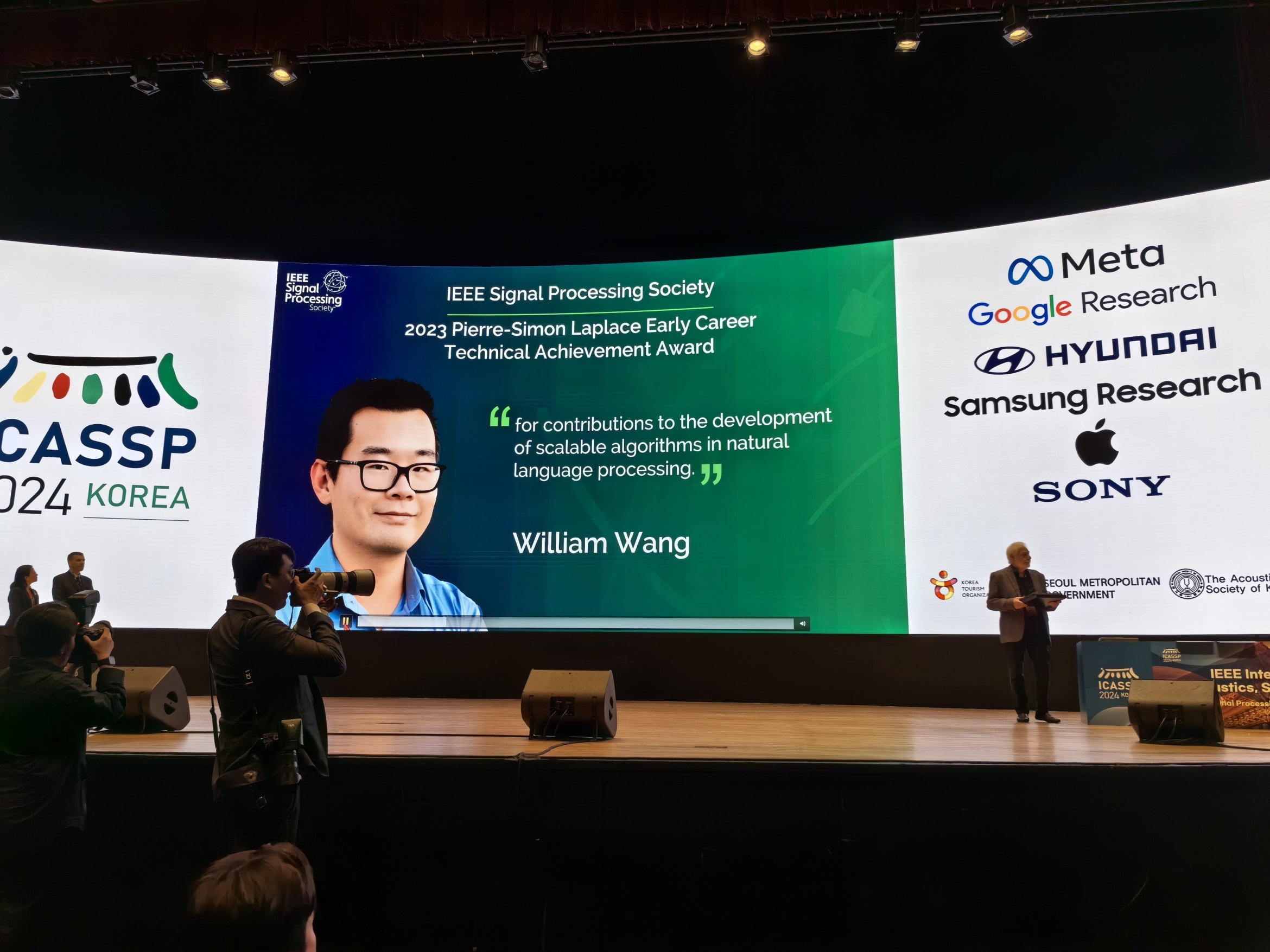
Computer scientist William Wang receives prestigious early career technical achievement award
As artificial intelligence continues to boom, scaling algorithms to ever-increasing data sets also becomes a bigger hurdle. Such is the case in the domain of natural language processing (NLP), or, the effort to get machines to understand and communicate with human language (think: ChatGPT, search engines and other text-based modalities).
“A key challenge in this domain is the tradeoff between scalability and accuracy,” said UC Santa Barbara computer scientist William Wang, who specializes in NLP. “While faster algorithms often compromise accuracy, more accurate models tend to be slower. Achieving a balance between these two aspects is critical yet challenging.” Thanks to the diversity of human expression, AI language models can often trip over things like ambiguity, slang, sarcasm, irony, translations, multiple meanings and other vagaries of human speech, or the models can take too long in interpreting them to be useful.
Wang’s considerable work to develop scalable algorithms that are both swift and accurate couldn’t be more necessary. And for his efforts, he has been awarded the Institute of Electrical and Electronics Engineers (IEEE) Signal Processing Society’s (SPS) Pierre-Simon Laplace Early Career Technical Achievement Award. He recently received this award in April, in Seoul, Korea.
“I’m extremely honored for this major award from IEEE SPS, and I have been a big fan of Laplace’s work,” said Wang, who was cited “for contributions to the development of scalable algorithms in natural language processing.” The award “honors an individual who, over a period of years in his/her early career, has made significant contributions to theory and/or practice in technical areas within the scope of the Society.”
Addressing problems in structured learning — in which the AI model is expected to predict multiple outputs per data input — has been a focal point of Wang’s research. “This is notably difficult due to the vast search space,” he said. The Wang Lab’s recent work with logic programs streamlines the process by utilizing in-context learning by large language models to enhance accuracy and reduce hallucinations (nonsensical outputs), without the need for further optimization algorithms. Another recent achievement involves an algorithm that accelerates the speed at which text-to-image models can predict output from novel data.
Laplace’s work — the 18th-19th century French scholar is known for among other things, advances within the realm of statistics and probability — plays a big role in Wang’s career, from creating constraints on data sets, to improving accuracy, to inferring variables based on observed data. The Wang research group’s recent paper at the NeurIPS 2023 conference uses Laplace’s signature Bayesian interpretation of probability to elucidate the emergent behavior of large language models.
Wang, who holds the campus’s Duncan and Suzanne Mellichamp Chair in Artificial Intelligence and Designs, and is also the director of UCSB Center for Responsible Machine Learning and of the UCSB NLP group, looks forward to further improving how AI can learn and interpret language.
“Scalable algorithms are vital for the advancement of AI,” he said. “Current state-of-the-art models in AI, including large language models and generative algorithms, are not optimally efficient in training and inference. Future AI development hinges on innovations in algorithms and architecture, promising more efficient training and inference processes for upcoming AI models.”
Sonia Fernandez
Senior Science Writer
(805) 893-4765
sonia.fernandez@ucsb.edu