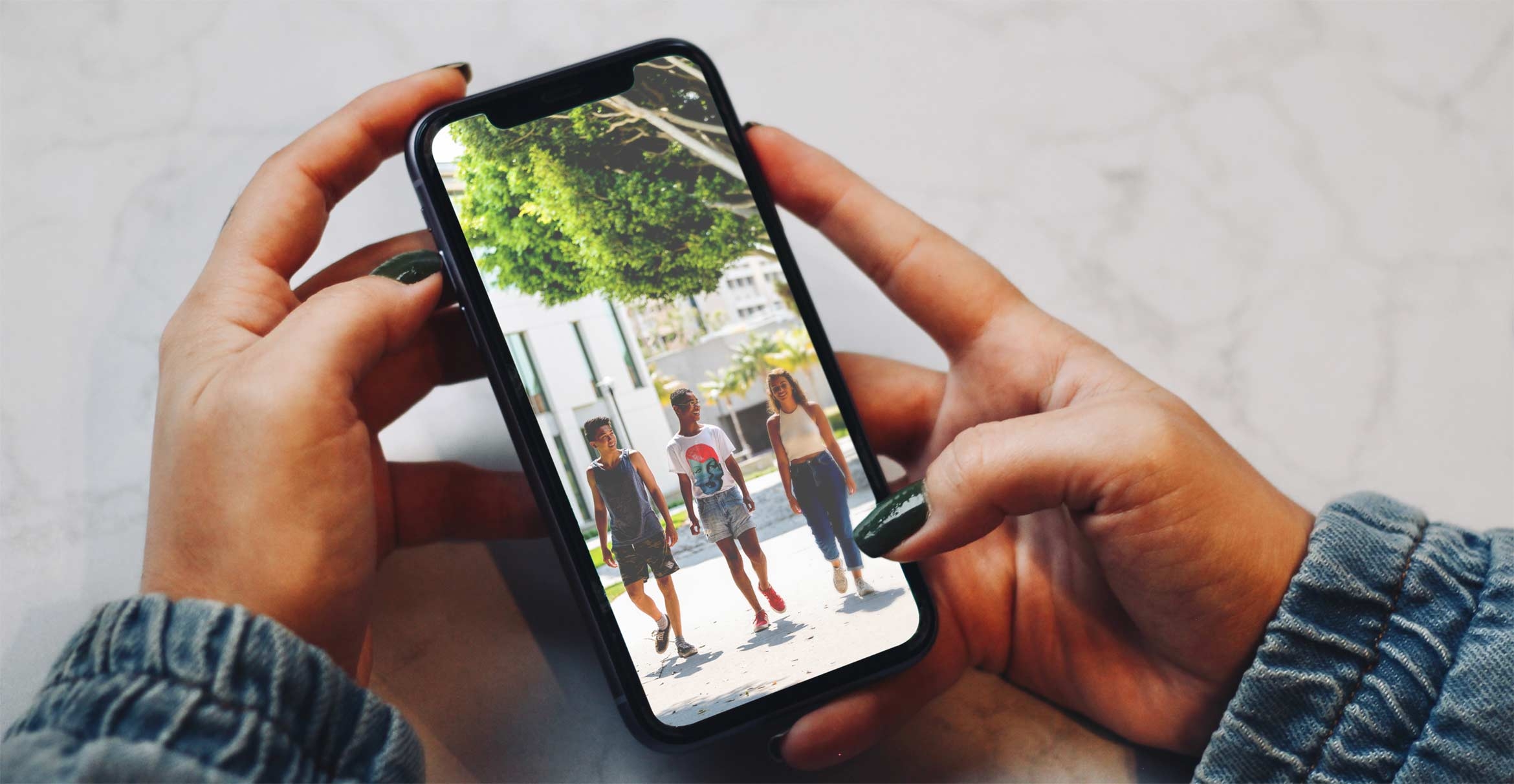
Computer vision and human annotations provide insights on inclusion in social media at scale
For many companies, diversity and inclusion aren’t just moral issues, they’re also matters of business. People like to see themselves in what they use and consume, so ensuring that your content matches the demographics of your market can prove to be an effective — even essential — way to bolster user engagement and retention.
With often robust metrics on user activity, social media provides an excellent case study. Researchers at UC Santa Barbara and University of Southern California (USC) investigated diversity, equity and inclusion across Snapchat. They found that content produced by the company reflected the diversity of the U.S. population. In fact, data showed, that racial minorities were slightly over represented. Content from partnered companies didn’t do as well, but fit with patterns in the broader entertainment industry.
The project spurred the creation of a sophisticated system to investigate representation in media, known as the Measuring and Tracking Inclusion Platform (MTI). The system shares features with other innovations from UCSB’s Media Neuroscience Lab, which takes a computational approach to communication research. The developers of MTI — Professor René Weber and researcher Musa Malik — hope that applying computer-assisted and fully automated techniques to inclusion research will make the practice more reliable, efficient, affordable and widespread. They document their findings and methodology in a research report that is available on the MTI platform.
“Understanding diversity and inclusion helps us recognize whose stories are being told, whose perspectives are being amplified and whose experiences are being marginalized,” explained Malik, a doctoral student in the Department of Communication and a researcher at the Media Neuroscience Lab. By gauging social participation, we can ask questions about whether our society is making use of its diverse population, or if it’s losing out on talent and productivity from individuals that are currently overlooked?
A unique opportunity
Snapchat’s parent company, Snap, approached researchers at UCSB’s Media Neuroscience Lab and USC’s Annenberg Inclusion Initiative to better understand their platform’s content. The company was curious whether the content it delivered represented its users — and the broader U.S. population — in terms of racial, gender and cultural diversity.
Snap provided the researchers with funding, a wealth of content data and academic freedom in publishing their findings. “Kudos to Snap,” said Weber, director and lead researcher of the Media Neuroscience Lab, noting, “They could have gone with a private company.” Instead, the media giant chose two academic groups well known and respected in this field.
Snapchat hosts three types of content: original content produced by Snap, partnered content produced by third parties and user-generated content. The scientists focused on the first two for privacy reasons.
The team approached their task with three techniques. They trained human “coders” to annotate gender, race, ethnicity, disability and LGBTQ identity in 300 shows of original content with 63 hosts and 726 speaking characters. They also integrated a bespoke computer vision system into the platform to analyze over 16,000 unique Snap stories randomly selected from content provided by the company. A subset of these automatically annotated stories were then validated with human coders.
Measuring inclusion patterns involves much more than simply looking at the characters on screen. Protocol matters. For instance, you need to decide who even counts as a character: Background characters? Individuals that don’t speak? Animations? Context matters, too. Are characters represented as central or peripheral characters in a narrative? Also critical: the choice of how to assign individuals to groups, and even which categories to include. Valid and reliable annotation protocols reduce personal bias and systematic error during coding.
The idea behind computer-assisted inclusion research is to take advantage of the strengths of both humans and computers to get better results than either could produce alone. “There are areas where computers are really good, and there are areas where a human may still have an edge,” Weber said. For instance, computer coding is reliable and scalable, while humans tend to have a better grasp of contextual information.
Weber and Malik designed their protocol based on the guide and expertise developed by their co-investigators at the USC Annenberg Inclusion Initiative. “It’s state of the art technology,” Malik said. “As far as we know, nobody else has implemented a system like ours anywhere.”
Trends in inclusion
The scientists found that diversity in Snap’s original content roughly matched the demography of the U.S. as a whole. Content hosts were 52.4% male and 47.6% female. LGBTQ representation (6.3%) was consistent with the broader U.S. population, at 7.1%. Meanwhile, more than half (57.1%) of hosts were from an underrepresented racial or ethnic group. This proportion grew to 61% when the team considered only speaking roles.
That said, men did have more speaking roles than women, at 60.5% versus 39.5%. And only a single host had a disability.
“Overall, Snapchat does really well in inclusion and diversity in Originals,” Weber said. The results suggest that putting resources toward their own content is a good strategy for inclusion and diversity. Other media companies could emulate their example, he added.
Partnered content did not achieve the same success as Snapchat’s own content, but the researchers said the content patterns were on par with the broader entertainment industry. For instance, 62.9% of characters were male, while only 37.1% were female (with less than 1% identified as non-binary). Meanwhile, 34.5% of individuals were from racial minorities. The contrast illustrates that inclusivity must be actively pursued.
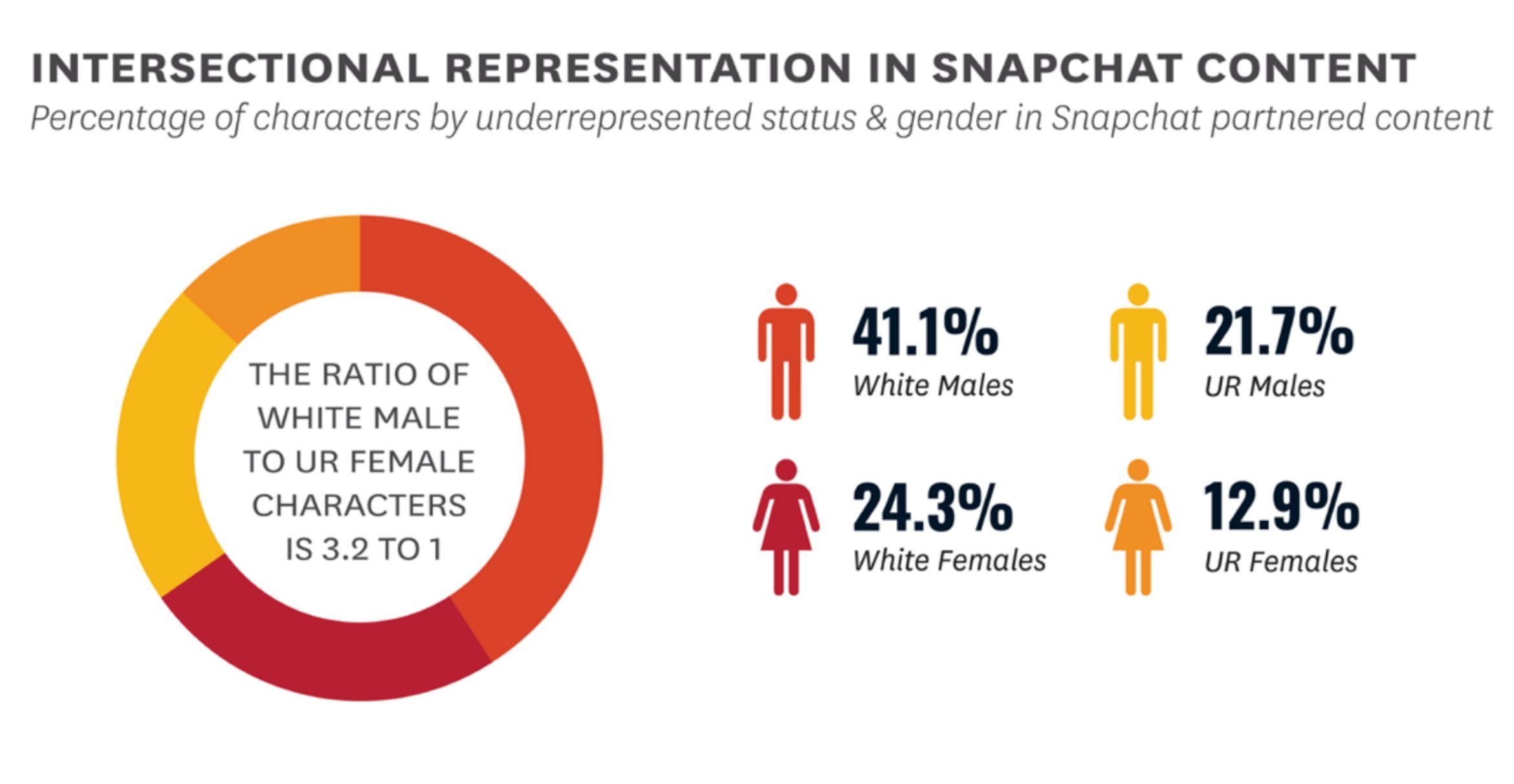
A new research platform
The researchers’ report featured numbers from human, computational hybrid annotation methods. Overall, the automated approach aligned very well with the human-based annotations. Incorporating computer-vision algorithms and machine learning will make the analysis of even larger content datasets faster and cheaper, as well as more comprehensive and reliable. It will enable more researchers, companies and organizations to analyze and monitor inclusion metrics.
The Measuring and Tracking Inclusion Platform brings content annotators and investigators together and standardizes the research process. It can handle all the logistics for the scientists and coders, train coders to be more reliable and visualize results for the different stakeholders.
MTI’s fully automated function can efficiently code massive content data sets. For instance, MTI uses visual features to identify and characterize individuals, a boon when not everyone depicted has a speaking role. But it is flexible; the system interprets spoken word for language related to the LGBTQ identity and monitors its prevalence. It can even automate the tedious task of cleaning and pre-processing data, like Snapchat’s nested stories, a feature the team hopes to generalize.
The platform simplifies many aspects of hybrid coding as well. All the small decisions involved in manual coding take up time and mental energy. Having the computer take care of tasks, like identifying which characters to count for the study, enables human coders to focus on the actual task of annotation, which results in more reliable content coding.
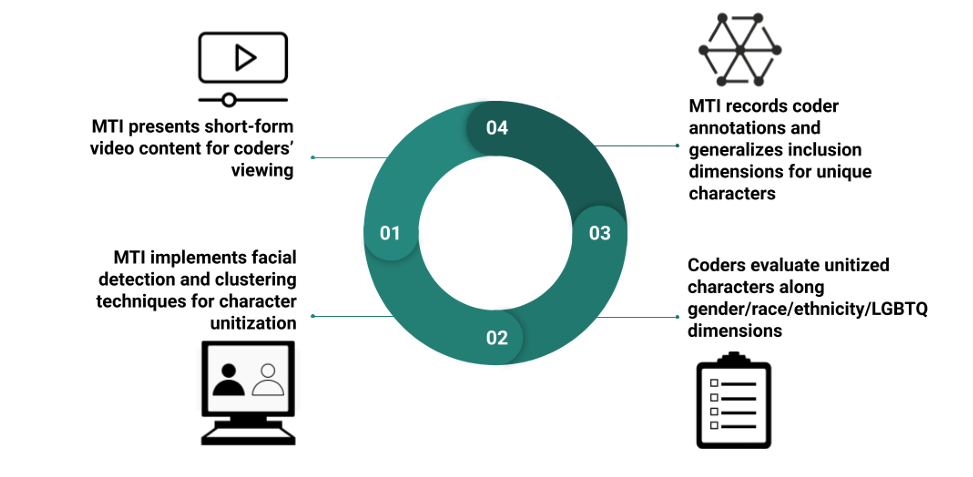
MTI also automatically tracks annotators’ reliability. For instance, it can determine if a particular coder always falls far outside of the rest of the team. The platform can then provide feedback to the investigator so they can re-train the individual and optimize the process. In the future, Weber and Malik plan to make this a real-time feature.
Still, Malik and Weber recognize the inherent limitations of their computational methods. “This is not a panacea for resolving inclusion issues,” Malik said. “But what we present is state of the art, it helps computer scientists build better pipelines; it helps inclusion scholars do better research; and it helps policy-makers design inclusive policy frameworks.”
The study’s findings add to the broader conversation around re-evaluating our assumptions in the media and entertainment industry. A previous collaboration between the Media Neuroscience Lab and Annenberg Inclusion Initiative considered how well movies with female and Black lead characters performed relative to those with white male leads. “If you control for production and advertising budgets,” Weber said, “we demonstrated that movies with Black and female leads are money makers, not money losers.”
The authors have a firm understanding of their role in this discussion. “We are not inclusion advocates,” Weber said. “We are scientists.” However, he believes bringing this matter to the forefront of scientific investigation is a valuable and important aim.
“It’s really problematic that this topic of inclusion has become politicized over the years,” Malik added. “And that’s where I think academics have a responsibility to do what they do best: solid, unbiased, and objective research.”
Harrison Tasoff
Science Writer
(805) 893-7220
harrisontasoff@ucsb.edu