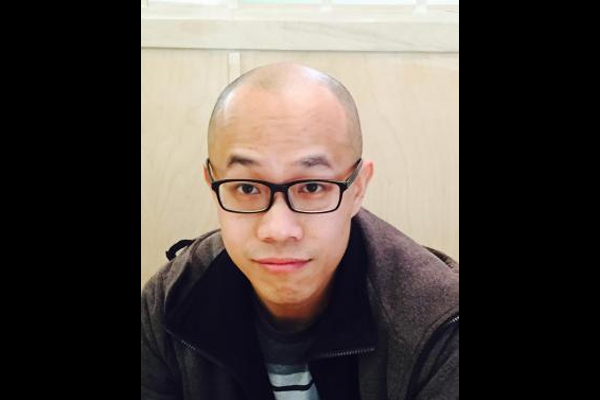
A World of Uncertainty
Between collecting data, conducting experiments and running simulations, uncertainty permeates science and engineering. So, understanding and accounting for uncertainty is an important aspect of modern research and development. This is precisely what Assistant Professor Mengyang Gu concerns himself with — developing methods to quantify uncertainty — in UC Santa Barbara’s Department of Statistics and Applied Probability.
The Society for Industrial and Applied Mathematics (SIAM) has announced Gu as the recipient of the 2022 SIAM Activity Group on Uncertainty Quantification Early Career Prize. The group awards this prize every two years to one individual in their early career for outstanding research contributions in the field of uncertainty quantification. The society cited Gu’s “contributions to the analysis and estimation of Gaussian process emulators” for their decision.
“The Department of Statistics & Applied Probability is very proud of Mengyang Gu and his well-deserved recognition,” said Department Chair Mike Ludkovski. “Since arriving at UCSB two years ago, Professor Gu has been on a very fast upward trajectory and is making numerous noteworthy contributions in applied statistics, as well as via interdisciplinary projects with geophysicists, chemical engineers and materials scientists. This prestigious award reflects his innovations in applying Gaussian-process emulators for uncertainty quantification in applied science domains.”
Given the prize’s exclusivity, Gu had no expectation of receiving it when he noticed the SIAM email in his inbox. He was delighted to learn he had been chosen, and believes he may be the first statistician to win the prize. “I’m very surprised, and I’m very happy,” he said. “I think they realized the importance of work from statisticians and data scientists.”
Uncertainty quantification finds its way into a diverse array of fields, including mathematics, statistics, physics and engineering. The goal of Gu’s work is to apply statistics to account for uncertainty in simulations and data processing in order to make these tasks faster, more robust and scalable to larger systems.
Gu points to density functional theory as an example. It’s currently one of the most useful methods for predicting a material’s properties based on its molecular configuration, he said. Unfortunately, the technique doesn’t translate well to large-scale systems. The computational complexity increases dramatically with a compound’s size, making the process prohibitively slow at a certain point. Gu is working to develop statistical models that approximate the physics simulation, expanding the complexity of molecules that researchers can simulate from first principles.
On the flip side, this approach can also help with data processing. Engineers that Gu works with at UCSB use microscopes to make observations and extract data from the videos they take. Unfortunately, processing these videos can be quite slow. Gu developed statistical models to quantify uncertainty from observations for data reduction and reconstruction, an innovation that accelerates data processing by roughly one hundred-fold.
These tasks are related, Gu explained, because after data is processed, researchers need to fit it with predictions from a scientific model, one that may be expensive to compute. “The two are like twin siblings: It’s the data and the model,” he said. “What we are doing is improving the performance of these techniques to make them robust and computationally feasible to apply to more complicated systems.”
Simplified models and faster data processing can have real world impacts. Gu collaborates with the U.S. Geological Survey, which uses satellite images and GPS to monitor volcanic activity, like the 2018 eruption of Kīlauea on the island of Hawai’i. “These physics models guide policy decisions and can save lives,” he said.
Gu compares the techniques he’s developing to the Kalman filter and fast Fourier transform, algorithms that are now standard tools in myriad fields. “These algorithms have become indispensable tools used widely in research and in daily life,” he said. Gu wants to make the data science techniques he’s working on as efficient, ubiquitous and easy to use as these staples.
“These high-performance tools should be available not only in certain research topics, but for everyone to use,” he said. “That’s the goal of our lab.”