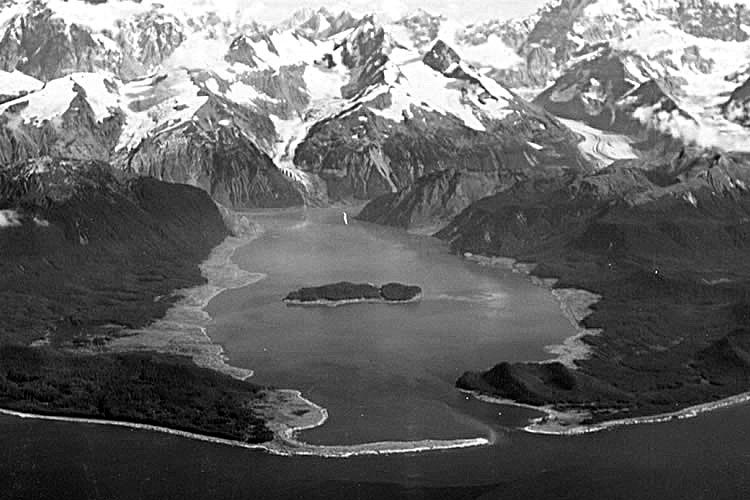
The Science of Tsunamis
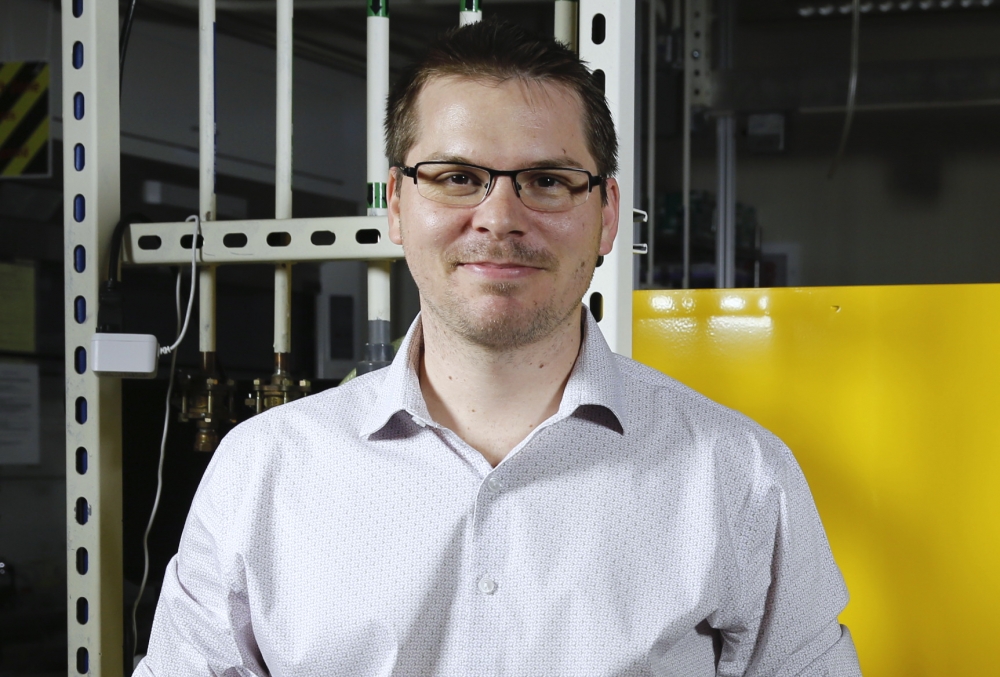
The word “tsunami” brings immediately to mind the havoc that can be wrought by these uniquely powerful waves. The tsunamis we hear about most often are caused by undersea earthquakes, and the waves they generate can travel at speeds of up to 250 miles per hour and reach tens of meters high when they make landfall and break. They can cause massive flooding and rapid widespread devastation in coastal areas, as happened in Southeast Asia in 2004 and in Japan in 2011.
But significant tsunamis can be caused by other events as well. The partial collapse of the volcano Anak Krakatau in Indonesia in 2018 caused a tsunami that killed more than 400 people. Large landslides, which send immense amounts of debris into the sea, also can cause tsunamis. Scientists naturally would like to know how and to what extent they might be able to predict the features of tsunamis under various circumstances.
Most models of tsunamis generated by landslides are based on the idea that the size and power of a tsunami is determined by the thickness, or depth, of the landslide and the speed of the “front” as it meets the water. In a paper titled “Nonlinear regimes of tsunami waves generated by a granular collapse,” published online in the Journal of Fluid Mechanics, UC Santa Barbara mechanical engineer Alban Sauret and his colleagues, Wladimir Sarlin, Cyprien Morize and Philippe Gondret at the Fluids, Automation and Thermal Systems (FAST) Laboratory at the University of Paris-Saclay and the French National Centre for Scientific Research (CNRS), shed more light on the subject. (The article also will appear in the journal’s July 25 print edition.)
This is the latest in a series of papers the team has published on environmental flows, and on tsunami waves generated by landslides in particular. Earlier this year, they showed that the velocity of a collapse — i.e., the rate at which the landslide is traveling when it enters the water — controls the amplitude, or vertical size, of the wave.
In their most recent experiments, the researchers carefully measured the volume of the granular material, which they then released, causing it to collapse as a cliff would, into a long, narrow channel filled with water. They found that while the density and diameter of the grains within a landslide had little effect on the amplitude of the wave, the total volume of the grains and the depth of the liquid played much more crucial roles.
A photograph of an experimental lab setup reflecting the fluid dynamics of a tsunami
Photo Credit: ILLUSTRATION COURTESY OF FAST AND UC SANTA BARBARA
“As the grains enter the water, they act as a piston, the horizontal force of which governs the formation of the wave, including its amplitude relative to the depth of the water,” said Sauret. (A remaining challenge is to understand what governs the speed of the piston.) “The experiments also showed that if we know the geometry of the initial column [the material that flows into the water] before it collapses and the depth of the water where it lands, we can predict the amplitude of the wave.”
The team can now add this element to the evolving model they have developed to couple the dynamics of the landslide and the generation of the tsunami. A particular challenge is to describe the transition from an initial dry landslide, when the particles are separated by air, to an underwater granular flow, when the water has an important impact on particle motion. As that occurs, the forces acting on the grains change drastically, affecting the velocity at which the front of grains that make up the landslide enters the water.
Currently, there is a large gap in the predictions of tsunamis based on simplified models that consider the field complexity (i.e., the geophysics) but do not capture the physics of the landslide as it enters the water. The researchers are now comparing the data from their model with data collected from real-life case studies to see if they correlate well and if any field elements might influence the results.