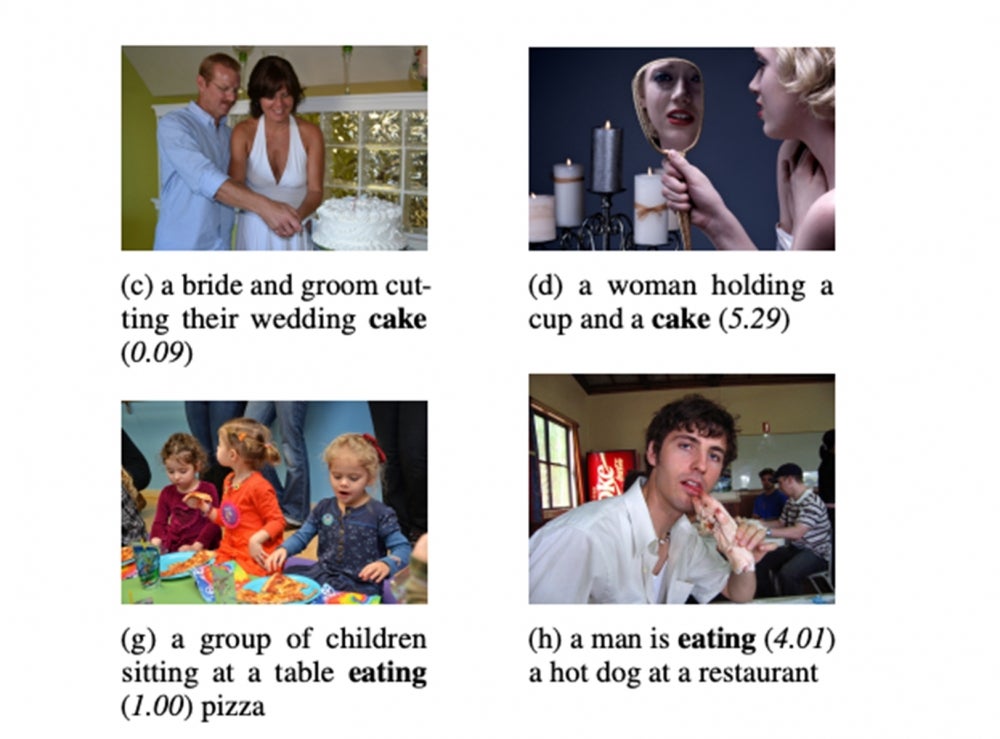
Words and Images
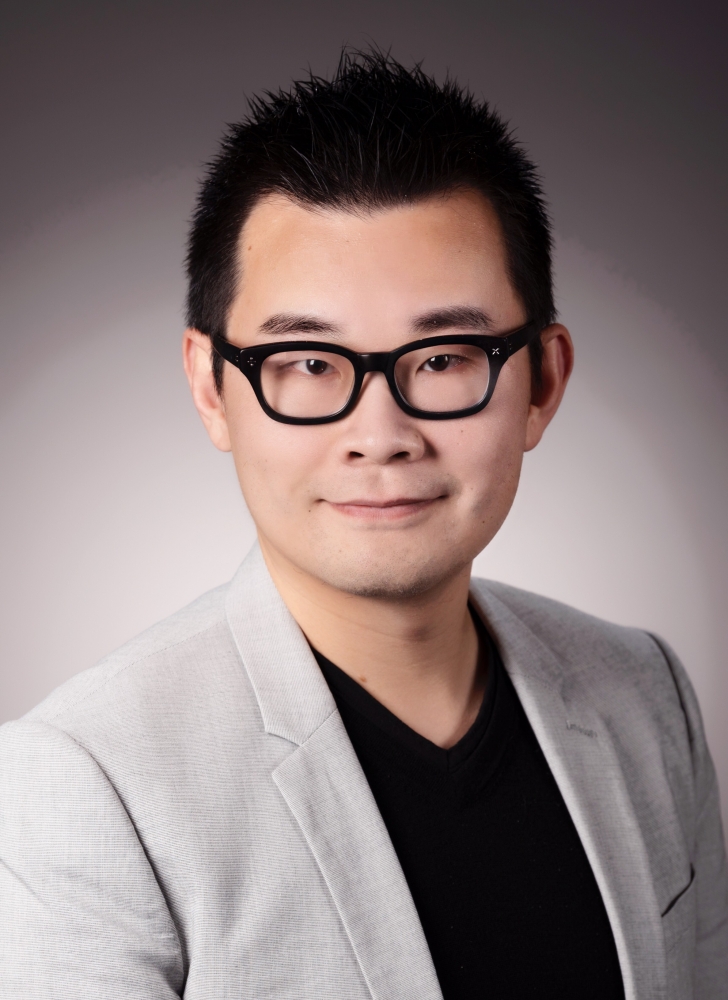
As we rely more on natural language processing to help us navigate our world, it’s more important than ever that these artificial intelligence models — used increasingly in applications such as caption generation for the visually impaired — remain true to reality.
“The issue is that deep learning-based neural language generation models have no guarantees in generating factually correct sentences that are faithful to the input data,” said UC Santa Barbara computer scientist William Wang. Over the many iterations it takes for a language generation model to learn how to describe or predict what a scene depicts, elements can creep in, causing phenomena such as errors in data-to-text translations or object hallucinations, in which the caption contains an object or an action that doesn’t exist in the image.
As a result, unless you have a way of reining in these errors (or you’re surrealist painter René Magritte) these mismatches could spell the end of the usefulness of the language generation model being used.
“This is a huge problem,” said Wang. “Imagine you are using a news summarization system to read earnings reports — the loss of faithfulness can give you wrong numbers, wrong facts and misinformation. Similarly, if a visually impaired person relies on an image captioning system to see the environment, wrong generation could create serious consequences.” Additionally, the quality and performance of subsequent language generation engines based on the outputs of faulty models will suffer significantly.
For his effort to create more robust deep learning-based natural language generation models, Wang has been chosen by the National Science Foundation to receive an Early CAREER Award for Faithful Natural Language Generation.
“We are extremely proud of Professor William Wang for this tremendous recognition,” said Rod Alferness, dean of the College of Engineering. “His work and leadership to push the boundaries in natural language processing and machine learning is critical to ensuring responsible and robust application of artificial intelligence to our daily life activities. We look forward to the exciting research results that will be enabled by this prestigious award from NSF.”
Wang’s CAREER award follows on the heels of his selection as a recipient of “The Future of AI: AI’s 10 to Watch” award from the Institute of Electrical and Electronics Engineers (IEEE) Intelligent Systems for his “contributions involving a hybrid mix of probabilistic programming, deep learning and natural language processing with applications to fake news.”
“I feel very lucky to receive these prestigious awards,” Wang said. “UCSB offers an open, friendly and interdisciplinary environment for faculty development, and I strongly believe it will become a global innovation hub for AI research in the very near future.”
The Search for the Truth
Wang’s research will involve investigations into the complex relationship between uncertainty and faithfulness, two important and sometimes opposing elements in the realm of deep learning.
“We believe that the AI model has to maintain a certain level of uncertainty in order to explore different solutions,” Wang said, “but it also has to be balanced and constrained at the same time.” The hypothesis is that too much uncertainty is bad, he explained: The systems will not know what to generate and it indicates very low confidence and potentially an unfaithful output. On the other hand, too little uncertainty could limit the AI’s ability to learn new things, he said, causing it to miss out on potential solutions.
Wang and his team will consider mitigation strategies to maintain an optimal balance, and build open-source software based on the emerging understanding of the faithfulness constraint. An additional component of his work on this project will be to bring artificial intelligence and natural language processing to underrepresented high school students.
“Ultimately, we want our research to go beyond analyzing static empirical data,” Wang added. “The current research in machine learning and AI primarily focus on independently and identically distributed data — each image is independent of one another. But how can we work with AI agents for dynamic decision making? This would be very practical for building AI agents that can interact with humans in the real world.”
A robust, faithful language generation model could improve existing technologies, such as dialog systems that can hold more nuanced, helpful conversations, or self-navigating agents that incorporate computer vision and natural language instructions. They can also open up possibilities in areas we haven’t yet imagined.
“There’s still a lot of work we need to do to improve the robustness of deep learning systems and faithfulness is a critical part of it,” Wang said.
Wang, who joined the UC Santa Barbara faculty in 2016, is an assistant professor in the Department of Computer Science. He holds the Duncan and Suzanne Mellichamp Chair in Artificial Intelligence and Designs, and directs both the UCSB NLP Group and the Center for Responsible Machine Learning. He is also the recipient of multiple faculty research awards since 2017, including three from IBM, two from Facebook, two from Google, and one each from Amazon, JP Morgan Chase, Adobe and Defense Advance Research Projects Agency (DARPA).