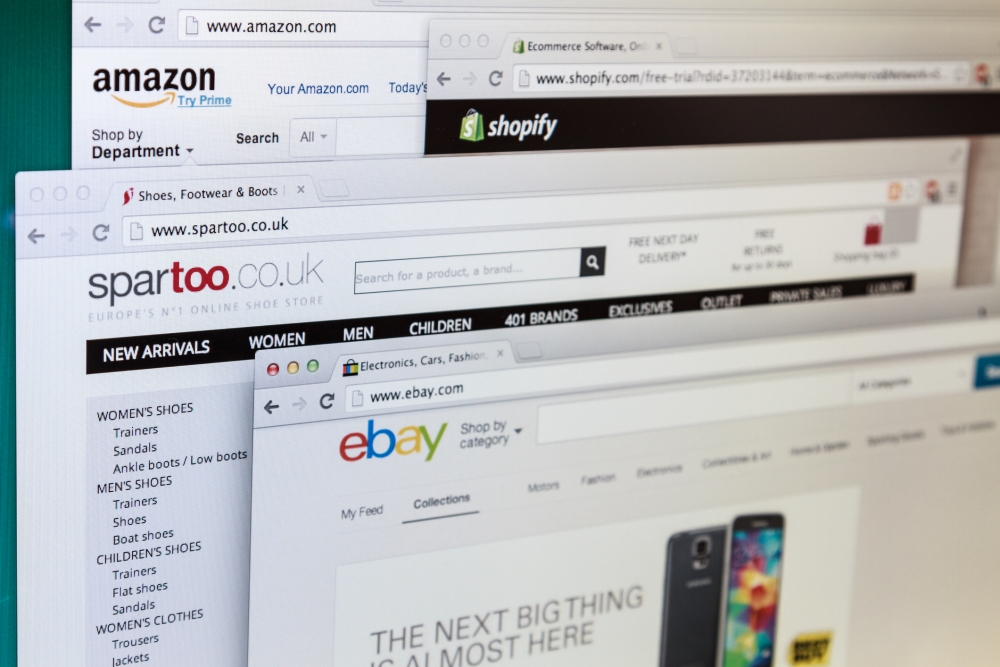
Recommended for You
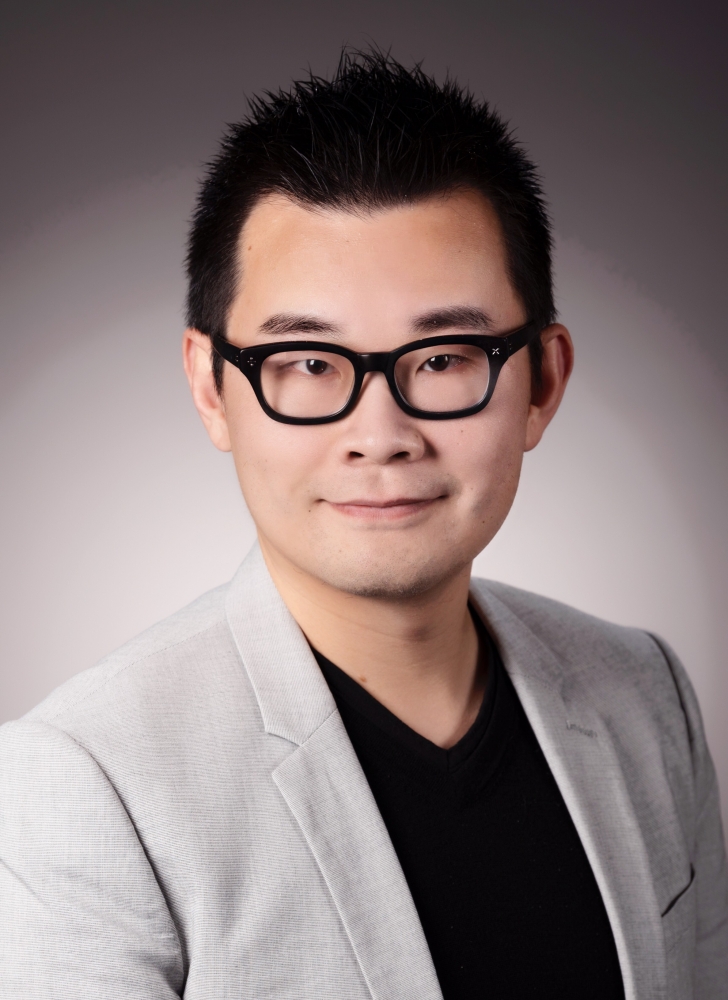
An emerging generation of artificial intelligence could get one step closer to passing the Turing test — a means of determining whether or not a computer is capable of thinking like a human being — all while helping you with your holiday shopping.
With $1 million in funding over three years from Google, a team of computer scientists that includes UC Santa Barbara’s William Wang hopes to gain insights that could lead to a more seamless conversation with online recommender dialog systems — a more interactive version of those AI-powered engines that help you steer toward products and services you could be interested in (think Amazon and Netflix).
“We hope to better understand users’ preferences via recommender dialogues,” said Wang, who joined the collaboration led by UC Santa Cruz computer science professor Lise Getoor. “In this project, we would like to learn the latent association of key entities in recommender dialogues and teach machines to reason and build better conversational recommender systems.”
Recommender dialogues, also known as conversational recommender systems, aim to go a step beyond conventional systems and engage the user in an interactive dialogue to elicit more details about their current interests and deliver more useful recommendations.
But existing dialogue agents have limited capabilities, according to Wang, who directs UCSB’s Natural Language Processing group and Center for Responsible Machine Learning.
“For example, if I say ‘I just had Italian for lunch; can you recommend a restaurant for dinner,’ a lot of dialogue systems don’t understand semantic constraints, so it might recommend a pizza place because of the implicit connections between ‘pizza,’ and ‘Italian’ food,” Wang said. “That’s an issue that is often frustrating for users.”
Wang, who specializes in natural language processing and knowledge representation, and Getoor, whose research interests include machine learning and reasoning under uncertainty, have joined forces with University of Southern California computer scientist Jay Pujara. His research in artificial intelligence emphasizes user utility.
“We form a strong tripod to support this project in terms of our backgrounds,” Getoor said. “We will also be working with leading experts at Google in machine learning, natural language processing and knowledge-based reasoning.”
The team’s approach will be based in probabilistic soft logic (PSL), an open-source toolkit developed by Getoor’s group at UC Santa Cruz that has been applied to a wide range of machine learning problems. PSL is a highly scalable probabilistic programming framework that is able to reason both statistically, using similarities, and logically, using soft rules.
“PSL is different from deep learning because of the way you can express soft tendencies, such as rules that apply most of the time but not all of the time, so it’s very intuitive and its results are easier to interpret,” Getoor said.
The researchers plan to extend PSL in key ways to integrate it with neural network-based deep learning approaches, which are highly popular but have some significant limitations. “The core of the project is figuring out how to integrate these approaches,” Getoor said. “What that will enable is a more informed dialogue that takes into account more of the user’s context.”
The project aims to improve the reasoning capabilities of dialogue agents, so the system can better understand a user’s request, using context and other information to generate a more appropriate response, Wang added.
The team also will explore a unified reasoning and discovery framework to design better algorithms and models for understanding the roles of context, knowledge and uncertainty in dialogues. In particular, they will investigate natural language understanding, knowledge discovery and reasoning, and natural language generation in task-oriented dialogues and beyond.