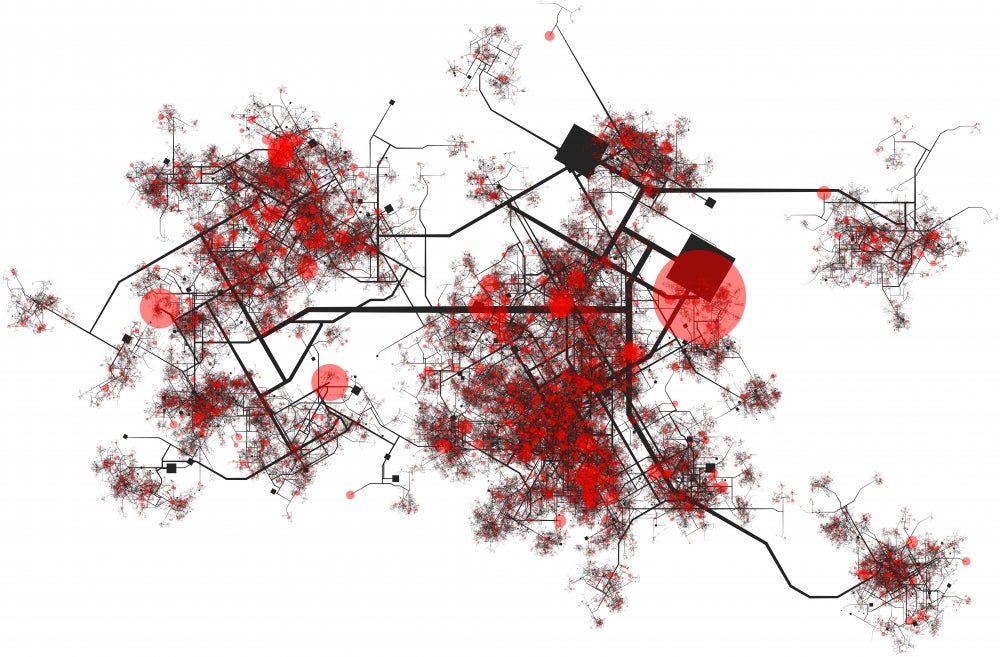
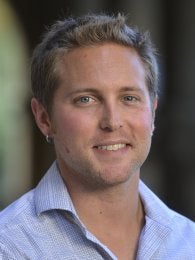
Models are only as good as the data they’re fed and the assumptions they’re based on. This can present a challenge when circumstances evolve rapidly in new directions.
As COVID-19 shutters cities and towns across the world, people aren’t behaving like epidemiologists are used to, and the models scientists would typically employ to predict the disease’s spread need to be revamped.
“Some of the early epidemiological models of COVID-19 rely on assumptions about human movement and connectivity that are totally unrealistic given that most of the country has been shut down,” said UC Santa Barbara disease ecologist Andy MacDonald.
MacDonald, UCSB postdoctoral researcher Dan Sousa, and their colleagues at Columbia and UC Berkeley just received a $200,000 grant from the National Science Foundation to address this issue. The funding comes from the foundation’s Early-Concept Grants for Exploratory Research (EAGER), which supports high risk, high reward proposals.
“These are ideas that may not pan out, but if they do, they have the potential to be transformative,” MacDonald said. “They often bring together expertise from multiple different disciplines.”
Classic epidemiological models use factors like commuting and air travel to estimate how connected different population centers are. But with people largely staying put, these data sources right now simply don’t provide much useful information.
The team plans to source more appropriate data to feed into the models, mostly using remote sensing. For instance, instead of using data like commuter travel to estimate connectivity, they’re looking at sources like night time lights, gridded population density and mobile phone density, location and movement. “All of these data sources will be brought together to create high resolution spatial networks of human population connectivity as well as to estimate where people are likely to be, interact and travel,” MacDonald said.
What’s more, many classic techniques simply don’t have high enough resolution to predict how the disease may spread at small scales, such as between neighborhoods, which can influence the likelihood of subsequent long-distance transmission. New data sources will provide information at a variety of spatial scales, giving scientists more detailed insights.
The higher resolution should also enable them to better estimate transmission dynamics as events unfold. “For instance, how quickly do we expect new cases to propagate out from hotspots in places that are opening up to places that are still closed,” MacDonald said.
Intending this to be an ongoing process, MacDonald, Sousa and their colleagues will continue to refine their techniques and share their results with the wider research community. The grant will support their work for one year, at which point they plan to apply for longer-term funding if the project proves successful, he said.