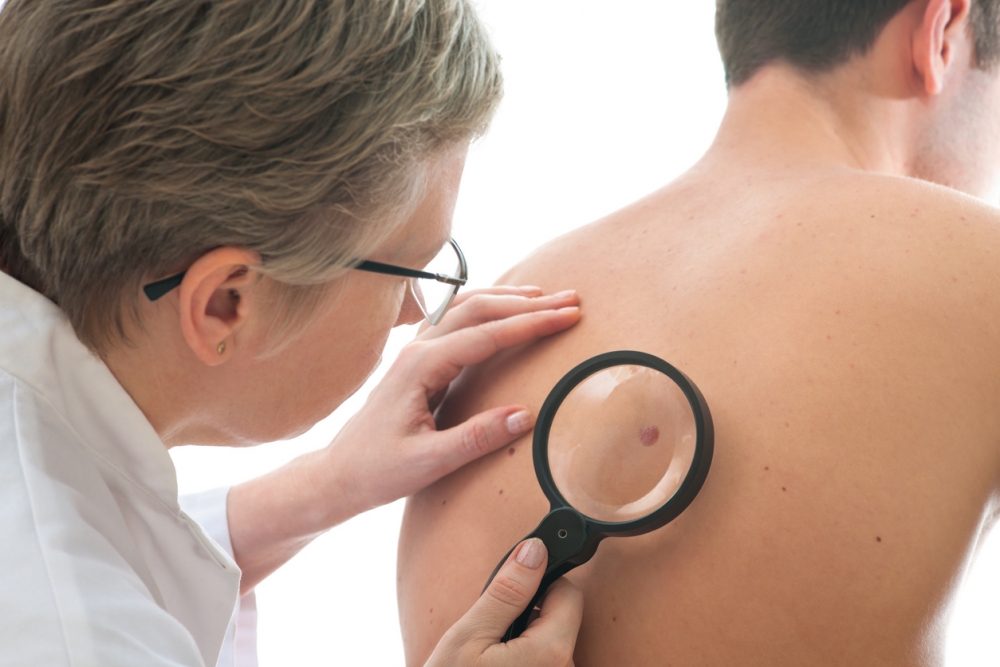
To Treat or Not to Treat
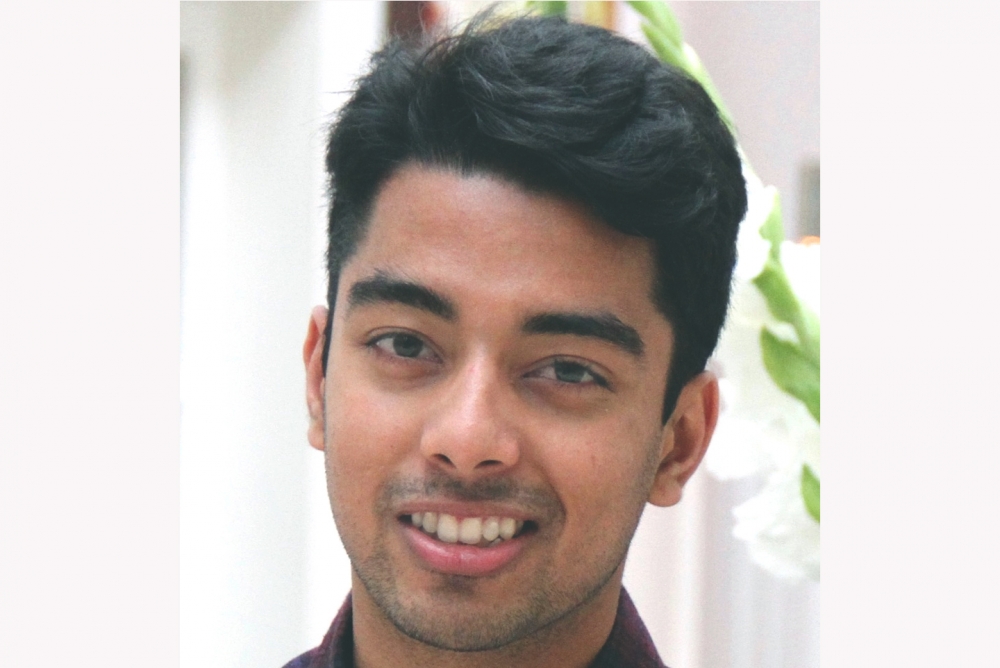
It’s a mark on your skin you’ve never noticed before. It’s a spot with irregular borders, or a mole that seems to be changing. Maybe it’s a wound that does not heal.
Is it melanoma? These days, the only way to be sure it’s not the relatively rare but potentially lethal skin cancer is to get a biopsy and consult a pathologist.
However, the poke and punch of traditional melanoma biopsies could, in the near future, be avoided. UC Santa Barbara undergrad Abhishek Bhattacharya is using the power of artificial intelligence to help people ascertain whether that new and strange mark is, in fact, the deadly skin cancer. Bhattacharya, a biology and computer science student in UCSB’s College of Creative Studies (CCS), has so far proven a 96 percent accuracy rating with his neural network model.
“We’re applying computer vision to solving medical problems,” said Bhattacharya, who, along with UC San Francisco (UCSF) physician and professor Dexter Hadley, developed the melanoma project and trained the neural network model to judge images of skin irregularities and predict whether the mark, mole or lesion is cause for concern.
Several factors make melanoma — the rarest form of skin cancer — difficult to detect. While skin cancer is the most common of all cancers, according to the American Cancer Society, melanoma accounts for only about one percent of skin cancers. Also, the disease can develop as a new mole, but it often develops over existing moles, and in some cases can be mistaken for scars and benign growths.
Additionally, other factors such as time constraints, priority of other illnesses and patient embarrassment frequently result in patients and doctors putting melanoma screening on the backburner. The result could be a dangerous oversight: Left unchecked, the tumor can spread to other parts of the body, including bones, organs and other tissue. Early detection is key to survival.
“What we’re trying to do is something humans can’t do,” said Dr. Hadley,” and humans can’t predict under-the-skin pathology from looking from the top.” Visual inspection is subjective, he said, even by physicians using a dermatoscope to see superficial and subsuperficial skin features and trained in the ABCDE (Area, Border, Color, Diameter, Evolution) protocol. However, the cancer is dangerous enough that when in doubt, physicians tend to err on the side of caution.
“You want to be oversensitive about this,” said Dr. Hadley, who specializes in precision medicine. For roughly every 30 excisions of suspicious moles and lesions, he estimated, one proves to be a melanoma.
And now machine learning can offer a better way. Taking advantage of major gains in computing power and big data — and inspired by how human brains work — scientists have figured out how neural network models, such as those that exist at the UCSF Institute for Computational Health Sciences, can be trained to predict whether a mole or lesion is melanoma based on images gathered from the world wide web.
“It’s an iterative process, essentially,” said Bhattacharya. Using loads of images scraped from the web, with labels indicating the type of skin lesions in those images, the neural network model learns what visual aspects are closely associated with melanoma diagnoses. This project uses a “convolutional neural network,” the architecture of which is modeled on an animal’s visual cortex.
“Basically an image is just an array of values, which tells it to be red or blue or green, for instance, and together you get an image,” Bhattacharya said. “And when a computer sees an image, it’s seeing a matrix of numbers.” As the model processes the image, different neurons on different layers are activated by various aspects of the image, in the same way that signals travel from neuron to neuron in our brains. Some lower-level neurons might be activated by edges and blobs of color, Bhattacharya said, but higher-level neurons could be more concerned with fuller concepts and classifications.
Like a human brain, the more practice the neural network model gets, the better it is at determining what the image might indicate. Fortunately, despite the rarity of melanomas — and hence relatively few images of them — the researchers were able to utilize “transfer learning.” With this method, the model was “pre-trained” in making fundamental recognitions and distinctions between images of everyday objects derived from a large repository called ImageNet, and then given medical images to inspect.
Three or so years ago, the researchers achieved roughly 85 percent accuracy with just a few hundred images. Since then, with more images and more refinement, the degree of accuracy has climbed to 96 percent — better than that of humans.
Still, Bhattacharya pointed out, the success rate “should be taken with a grain of salt,” as it still needs to be proven within a clinical setting. While a head-to-head test between the neural network model and a dermatologist looking at images might prove the model the winner, its efficacy in a real-world medical scenario is yet to be demonstrated.
“We really want to get this into the hands of clinicians,” Bhattacharya said. As the research team works to make that happen, Bhattacharya, Hadley and other physicians have teamed up to launch a company called SkinIQ, aimed at bringing artificial intelligence to skin cancer detection. The team hopes to create a mobile app that will allow people — especially those who live in remote areas with little access to skin experts — to make their own assessments as to whether they may or may not have melanoma.
The soon-to-be college senior has also made presentations and garnered awards and recognition for his work, including a second place-ranked paper at a meeting of the American Medical Informatics Association in San Francisco last March. In 2016 he received honorable mention and was the only UCSB representative at the annual UC Berkeley Big Ideas Competition. His work has contributed to numerous successful academic grants, including the prestigious and inaugural UCSF Marcus Program in Precision Medicine Initiative.
As Bhattacharya enters his senior year at UCSB, with medical school on the horizon, he points to the College of Creative Studies as setting him up for success. In particular, he credits his CCS faculty advisor Phill Conrad and research mentor Linda Petzold (a professor in the departments of computer science and mechanical engineering) for enabling his entry into translational medicine and precision medicine — emerging fields in which data, technology and innovation propel rapid developments and progress in the treatment and cure of diseases.
Bhattacharya will continue to work in collaboration with Dr. Hadley at UCSF’s Institute for Computational Health Sciences at the intersection of medical and data science, but he attributes his solid foundation to CCS.
“CCS has really been great,” he said, adding that the idea for the use of the neural network for medicine was born at UCSB. “When I came here, I knew I wanted to do biology and computer science. Those are my loves.”